Tối ưu hóa đa mục tiêu quá trình khoan xung cho vật liệu làm khuôn để nâng cao năng suất và chất lượng lỗ gia công
TÓM TẮT
Nâng cao các chỉ tiêu kỹ thuật của quá trình khoan xung là một giải pháp hiệu quả để giảm chi
phí sản xuất. Nghiên cứu này đề cập đến bài toán tối ưu hóa đa mục tiêu để nâng cao tốc độ bóc
tách vật liệu và giảm độ mở rộng của lỗ. Các thông số công nghệ bao gồm cường độ dòng điện, độ
kéo dài xung, khoảng cách xung và hệ số điều chỉnh điện áp. Quá trình thực nghiệm được tiến hành
trên máy khoan xung EDD theo ma trận quy hoạch Box-Behnken. Mối quan hệ phi tuyến tính giữa
các thông số công nghệ và hàm mục tiêu được xây dựng thông qua mô hình Kriging. Cuối cùng, một
thuật toán di truyền vi mô dựa trên kho lưu trữ (AMGA) đã được sử dụng để giải quyết mối tương
quan giữa các yếu tố đầu ra xác định các thông số tối ưu. Kết quả cho thấy, độ mở rộng của lỗ có thể
giảm khoảng 12,26%, trong khi tốc độ bóc tách vật liệu được cải thiện khoảng 32,17%. Sự kết hợp
giữa mô hình Kriging và AMGA có thể được coi là một cách tiếp cận thông minh để mô hình hóa các
quy trình khoan xung và tạo ra kết quả tối ưu đáng tin cậy.
Tóm tắt nội dung tài liệu: Tối ưu hóa đa mục tiêu quá trình khoan xung cho vật liệu làm khuôn để nâng cao năng suất và chất lượng lỗ gia công
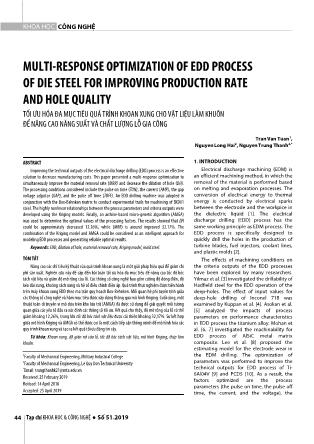
CÔNG NGHỆ Tạp chí KHOA HỌC & CÔNG NGHỆ ● Số 51.2019 44 KHOA HỌC MULTI-RESPONSE OPTIMIZATION OF EDD PROCESS OF DIE STEEL FOR IMPROVING PRODUCTION RATE AND HOLE QUALITY TỐI ƯU HÓA ĐA MỤC TIÊU QUÁ TRÌNH KHOAN XUNG CHO VẬT LIỆU LÀM KHUÔN ĐỂ NÂNG CAO NĂNG SUẤT VÀ CHẤT LƯỢNG LỖ GIA CÔNG Tran Van Tuan1, Nguyen Long Hai2, Nguyen Trung Thanh2,* 1. INTRODUCTION Electrical discharge machining (EDM) is an efficient machining method, in which the removal of the material is performed based on melting and evaporation processes. The conversion of electrical energy to thermal energy is conducted by electrical sparks between the electrode and the workpice in the dielectric liquid [1]. The electrical discharge drilling (EDD) process has the same working principle as EDM process. The EDD process is specifically designed to quickly drill the holes in the production of turbine blades, fuel injectors, coolant lines, and plastic molds [2]. The effects of machining conditions on the criteria outputs of the EDD processes have been explored by many researchers. Yılmaz et al. [3] investigated the drillability of Hadfield steel for the EDD operation of the deep-holes. The effect of input values for deep-hole drilling of Inconel 718 was examined by Kuppan et al. [4]. Asokan et al. [5] analyzed the impacts of process parameters on performance characteristics in EDD process the titanium alloy. Mohan et al. [6, 7] investigated the machinability for EDD process of AlSiC metal matrix composite. Lee et al. [8] proposed the estimating model for the electrode wear in the EDM drilling. The optimization of parameters was performed to improve the technical outputs for EDD process of Ti- 6Al04V [9] and PCDS [10]. As a result, the factors optimized are the process parameters (the pulse on time, the pulse off time, the current, and the voltage), the ABSTRACT Improving the technical outputs of the electrical discharge drilling (EDD) process is an effective solution to decrease manufacturing costs. This paper presented a multi-response optimization to simultaneously improve the material removal rate (MRR) and decrease the dilation of hole (DH). The processing conditions considered include the pulse on time (TON), the current (AMP), the gap voltage adjustor (GAP), and the pulse off time (TOFF). An EDD drilling machine was adopted in conjunction with the Box-Behnken matrix to conduct experimental trails for machining of SKD61 steel. The highly nonlinear relationships between the process parameters and criteria outputs were developed using the Kriging models. Finally, an archive-based micro-genetic algorithm (AMGA) was used to determine the optimal values of the processing factors. The results showed that DH could be approximately decreased 12.26%, while (MRR) is around improved 32.17%. The combination of the Kriging model and AMGA could be considered as an intelligent approach for modeling EDD processes and generating reliable optimal results. Keywords: EDD, dilation of hole, material removal rate, Kriging model, mold steel. TÓM TẮT Nâng cao các chỉ tiêu kỹ thuật của quá trình khoan xung là một giải pháp hiệu quả để giảm chi phí sản xuất. Nghiên cứu này đề cập đến bài toán tối ưu hóa đa mục tiêu để nâng cao tốc độ bóc tách vật liệu và giảm độ mở rộng của lỗ. Các thông số công nghệ bao gồm cường độ dòng điện, độ kéo dài xung, khoảng cách xung và hệ số điều chỉnh điện áp. Quá trình thực nghiệm được tiến hành trên máy khoan xung EDD theo ma trận quy hoạch Box-Behnken. Mối quan hệ phi tuyến tính giữa các thông số công nghệ và hàm mục tiêu được xây dựng thông qua mô hình Kriging. Cuối cùng, một thuật toán di truyền vi mô dựa trên kho lưu trữ (AMGA) đã được sử dụng để giải quyết mối tương quan giữa các yếu tố đầu ra xác định các thông số tối ưu. Kết quả cho thấy, độ mở rộng của lỗ có thể giảm khoảng 12,26%, trong khi tốc độ bóc tách vật liệu được cải thiện khoảng 32,17%. Sự kết hợp giữa mô hình Kriging và AMGA có thể được coi là một cách tiếp cận thông minh để mô hình hóa các quy trình khoan xung và tạo ra kết quả tối ưu đáng tin cậy. Từ khóa: Khoan xung, độ giãn nở của lỗ, tốc độ bóc tách vật liệu, mô hình Kriging, thép làm khuôn. 1Faculty of Mechanical Engineering, Military Industrial College 2Faculty of Mechanical Engineering, Le Quy Don Technical University *Email: trungthanhk21@mta.edu.vn Received: 25 February 2019 Revised: 14 April 2018 Accepted: 25 April 2019 SCIENCE TECHNOLOGY Số 51.2019 ● Tạp chí KHOA HỌC & CÔNG NGHỆ 45 electrode characteristics (the geometry and the material), dielectric properties (type, dielectric strength, and viscosity), and the workpiece properties. The technological responses considered are surface roughness properties and the hole quality. In the current work, a multiple-response optimization of process parameters for the EDD process of the die material has performed to improve the MRR and reduce the DH. The inputs may have complicated impacts on the outputs and an effective approach for modeling EDD process behavior and the optimizing processing factors in terms of improving working performances still is a significant contribution. 2. MATERIALS AND METHODS 2.1. Optimization framework The procedure for generating optimal values is shown in Fig. 1. The experimental matrix generated by the Box- Behnken method is applied to save the experimental costs [11, 12]. Process parameters, including the AMP, TON, TOFF and GAP as well as three levels (-1; 0; +1) were shown in Table 1. The chemical compositions of SKD61 material are shown in Table 2. The parameter ranges are chosen based on the machine tool’s characteristics, the recommendations of the electrode’s manufacturer, and material properties. The predictive models of DH and MRR are then developed with respect to process parameters using the experimental data. In this paper, the AMGA is applied to find a set of feasible solutions, which can be used to enhance surface integrity. AMGA is an evolutionary optimizing technique, in which each response is resolved individually and a set of feasible solutions is observed. The operating mutation and solution are performed by means of the chosen designs. The search history and solution selection are conducted using a myriad of different heuristics. The best values of the responses were determined at the end of the convergent run. Many researchers indicated that AMGA possesses high computational efficiency and provides the globally optimal solutions, as compared to other optimization algorithms [13, 14]. Figure 1. Systematic optimization procedure Table 1. Process parameters and their levels Symbol Parameters level -1 level 0 level +1 AMP Current (A) 2 5 8 TON Pulse on time (µs) 40 90 140 GAP Gap voltage adjustor 2 5 8 TOFF Pulse off time (µs) 15 65 115 Table 2. Chemical compositions of SKD61 C Si Mn P S Cr Mo Cu V 0.38 0.9 0.28 0.03 0.02 4.9 1.2 0.26 0.95 2.2. Experiments and measurements The CNC EDM machine, namely MAX S.E.E S36 is used to perform the experimental runs as depicted in Fig. 2. The brass electrode of 1 mm diameter and 400 mm length is used as tool material. The vertical axis movement of the electrode is controlled using the servo motor and the desired depth of 10 mm is set for each run. The debris is removed with the aid of the fluid. The holes are drilled on the workpiece having the dimensions of 20 x 10 x 100 mm. All faces of the specimens are ground and polished before the EDM drillings. The workpiece having the mating interface is used to measure the length of the drilling hole. The DH is an indicator of the machined part quality. The diameter of each hole is measured at different positions and the average value (ΔD) is calculated to find the mean diameter of the hole at the top as well as the bottom. The value of DH is estimated using the following equation, as depicted in Fig. 2: eDH D D= (1) where De is the original diameter of the electrode. ΔD is the circularity of drilled hole and calculated using Eq. (2): ....1 2 nD D DD n + + + = (2) where n is the number of measuring points. The machined holes are dried, cleansed, scanned using a 3D high-resolution scanner, namely 450V. The captured images are used to the diameters and the length of each hole. Generally, the MRR is generally considered as an important indicator of the production rate. The MRR (mm3/s) is calculated using the following equation: ( )22 top bottoma a D D LV D LMRR t 4t 16t + = = = (3) where Da (mm), L (mm), and t (s) are the average diameter of the hole, the length of the hole, and machining time, respectively. ΔDtop and ΔDbottom are the top and bottom circularity of each hole, respectively. CÔNG NGHỆ Tạp chí KHOA HỌC & CÔNG NGHỆ ● Số 51.2019 46 KHOA HỌC 2.3. Kriging Model The Kriging models of DH and MRR in terms of processing factors are proposed using the experimental data. It can be described by means of equation 4: ( ) ( ) ( )y x p x z x= + (4) where y(x) denotes the polynomial function to be developed, p(x) presents a known polynomial function and z(x) is the realization of a normally distributed stochastic process [15]. The Kriging predictor at a specific value of x is calculated by: (x) ( ) ( )T 1y β r x R f pβ = + (5) where f denotes the column vector containing the sample data and p presents the filled column vector. The factor β is calculated using the following equation: ( )T 1 1 T 1yβ p R p p R = (6) rT(x) is the correlation vector and estimated by: T 1 2 N Tr (x) [R(x,x ),R(x,x ),...,R(x,x )]= (7) In this paper, the Gaussian correlative function is used and defined as: m i j 2 k k k k 1 R(θ) exp[ θ (x x ) ] = = (8) The estimated variance of the proposed model is calculated by: 12 (y pβ)R (y pβ)σ N = (9) The correlation factor θk is estimated by: 2 k [Nln(σ ln R ]max (θ ) 2 + = (10) where 2 σ and R are the function of θk. Figure 2. Experiments 3. RESULTS AND DISCUSSION 3.1. Development of Kriging models The DOE matrix and experimental results of the EDD process are given in Table 3. For constructing a Kriging surrogate, it is necessary to obtain the unknown correlation parameter θk and scalar factor β in Eqs. 6 & 10. The correlation parameter θk and scalar factor β were observed using the maximum likelihood method, as shown in Table 4. Table 3. Experimental results No. AMP (A) TON (µs) TOFF (µs) GAP DH (mm) MRR (mm3/s) 1 5 90 65 5 0.601 0.5400 2 2 90 65 2 0.392 0.4651 3 5 90 15 2 0.513 0.3515 4 5 90 65 5 0.604 0.5588 5 5 90 115 8 0.599 0.8867 6 5 90 115 2 0.346 0.2005 7 5 140 65 8 0.824 1.5965 8 2 90 15 5 0.611 0.3046 9 5 40 115 5 0.352 0.1806 10 8 90 115 5 0.674 0.9454 11 5 40 65 2 0.291 0.1688 12 5 90 65 5 0.603 0.5478 13 2 90 65 8 0.726 0.5319 14 8 90 65 8 0.986 2.6267 15 2 40 65 5 0.322 0.1289 16 8 140 65 5 0.891 1.5989 17 5 90 15 8 0.848 1.4265 18 2 90 115 5 0.321 0.1198 19 8 90 15 5 0.925 1.3470 20 5 40 65 8 0.691 0.7566 21 8 90 65 2 0.776 0.7113 22 5 140 115 5 0.479 0.4506 23 2 140 65 5 0.574 0.3242 24 5 140 15 5 0.802 0.8073 25 5 90 65 5 0.608 0.5531 26 8 40 65 5 0.664 0.8438 27 5 40 15 5 0.461 0.3541 28 5 140 65 2 0.601 0.3982 29 5 90 65 5 0.607 0.5393 Table 4. The parameters of the Kriging models Responses Correlation parameter θk Scalar factor β AMP GAP TON TOFF DH 0.124259 0.118648 0.105811 0.121930 0.014445 MRR 0.217231 0.204221 0.071491 0.060502 0.127774 SCIENCE TECHNOLOGY Số 51.2019 ● Tạp chí KHOA HỌC & CÔNG NGHỆ 47 (a) For the dilation of hole (b) For the material removal rate Figure 3. Investigations of the model accuracy for the Kriging models 3.2. Model fitness The adequacy of the Kriging models can be evaluated using the R2-values. The R2-values of DH and MRR are 0.9886 and 0.9874, respectively. Additionally, the observed data distributed on the straight lines, as exhibited in Fig. 3. It can be stated that there is a good agreement between predicted and measured values. Therefore, the fidelity of the Kriging models proposed for the machining responses is acceptable. 3.3. The effects of process parameters on the technical responses The effects of processing factors on the DH are shown in Figs. 4a and b. At a higher value of AMP, GAP, and TON, the discharge energy will increase, leading to excessive material removal, which results in larger dimensions. In contrast, the decreased DH is associated with an increased TOFF. The longer the TOFF, the smaller discharge energy becomes, which decreases the evaporating of materials, leading to smaller diameters. The effects of processing factors on the material removal rate are shown in Figs. 4 c and d. An increase in the AMP, GAP, and TON causes higher discharge energy, which leads to an increment in the rate of melting and evaporation. A higher pulse off time decreases the discharge energy, leading to a slower MRR. The contributions of inputs are depicted using Pareto charts, as shown in Fig. 5. The blue bar shows that the process parameters have a positive effect on the objective, while the red denotes a negative influence. As a result, the percentage contributions of AMP, GAP, TOFF, and TON are 17.90%, 16.44%, 14.15%, and 13.89%, respectively. The AMP 2 account for the highest percentage contribution with respect to quadratic terms (7.20%); this followed by TOFF2 (4.90%), TON2 (4.63%), and GAP2 (3.70%). The contributions of the interaction terms, including GAP-TON and GAP-TOFF are 4.60% and 2.13%, respectively, which have a negative effect on the dilation of hole. As shown in Fig. 5b, the AMP has the largest effect on MRR with a percentage of 14.92%, followed by GAP (11.95%), TON (9.20%), and TOFF (7.47%). The GAP2 is the most affected factor due to the highest contribution (9.28%) with regard to the quadratic term, followed by AMP2 (8.51%). (a) DH versus AMP and GAP (b) DH versus TON and TOFF CÔNG NGHỆ Tạp chí KHOA HỌC & CÔNG NGHỆ ● Số 51.2019 48 KHOA HỌC (c) MRR versus AMP and GAP (d) MRR versus TON and TOFF Figure 4. Interaction effects of each machining parameters on the objectives (a) Pareto chart for DH (b) Pareto chart for AMRR Figure 5. Pareto charts for the objectives 4. OPTIMIZATION RESULTS The developed models for the DH and MRR are optimized using AMGA, which has the capacity of finding the optimal solution of a multi-objective problem. It is tough work to determine the optimal process parameters for simultaneous improving machining responses. Additionally, processing factors have complex effects on the technical outputs. The optimizing issue can be described as follows: Find X = [AMP, TON, TOFF, GAP] Maximize MRR; Minimize DH 2 ≤ AMP ≤ 8 (A), 40 ≤ TON ≤ 140 (µs), 15 ≤ TOFF ≤ 115 (µs), 2 ≤ GAP ≤ 8. Figure 6. Pareto fonts generated by AMGA SCIENCE TECHNOLOGY Số 51.2019 ● Tạp chí KHOA HỌC & CÔNG NGHỆ 49 Table 5. Optimization results Method Optimization parameters Responses AMP (A) TON (µs) GAP TOFF (µs) DH (mm) MRR (mm3/s) AMGA 6 139 6 114 0.5273 0.7961 Initial 5 90 5 65 0.6010 0.5400 Improvement (%) -12.26 32.17 The developed equations showing the relationship between process parameters and machining responses are used to find optimal parameters by means of the AMGA. The operating values of AMGA parameters, including the population size, number of generations, crossover probability, crossover distribution index, and mutation distribution index, are 20, 40, 0.9, 10, and 20, respectively. The Pareto front generated by the AMGA algorithm was exhibited in Fig. 6, in which the pink points are feasible solutions. The optimization results are listed in Table 5. The dilation of the hole is decreased around 12.26% and the material removal rate is approximately increased 32.17%. 5. CONCLUSION This paper presented a machining parameters-based optimization for the electrical discharge drilling of SKD61 material in order to decrease the DH and the MRR. The Kriging models of two responses were developed in terms of processing factors, including the TON, the AMP, the GAP, and the TOFF. An AMGA was used to predict the optimal values. The conclusions of this research can be listed as follows: 1. The predictive models for the dilation of the hole and the material removal rate having R²-values of 0.9886 and 0.9874, respectively indicate a good correlation between the predicted and experimental values. The models proposed are effectively exhibited the nonlinear relationships in terms of process parameters. The predictive models developed can be used for the electrical discharge drilling process of SKD61 material to forecast the optimal process parameters with the acceptable accuracy. 2. The Pareto fronts generated by the AMGA can significantly support the EDD operators to select appropriate parameters to decrease the dilation of the hole and increase the material removal rate. The selection of optimal parameters can decrease the efforts required and machining costs as well as machining time. 3. The hybrid approach consisting the Kriging models and AMGA can widely apply for the optimization of the electrical discharge drilling process instead of using practical experience and operating guide. The method approach in this research is multi-purposeful and can be used in all cases of electrical discharge drilling processes with different materials. REFERENCES [1]. Rajurkar KP., Levy G., Malshe A., Sundaram MM., McGeough J.,DeSilva A., Hu X., Resnick R., 2006. Micro and nano machining by electro-physical and chemical processes. Ann CIRP, 55/2, 643–666. [2]. Vijay C., 2003. Performance characteristics of electro discharge drilling process. MSc thesis, Industrial and Management Systems Engineering, University of Nebraska–Lincoln, 1–30. [3]. Yılmaz V., Sarıkaya M., Dilipak H., 2015. Deep micro-hole drilling for Hadfield steel by electro-discharge machining (EDM). Mater Technol, 49/3, 377– 386. [4]. Kuppan P., Rajadurai A., Narayanan S., 2008. Influence of EDM process parameters in deep hole drilling of Inconel 718. Int J Adv Manuf Technol, 38/1-2, 74–84. [5]. Asokan T., Reddy SS., Costa PDE., 2000. Electrical discharge drilling of titanium alloys for aerospace applications. Proceedings of 19th AIMTDR conference. IIT Madras, Chennai, 161–165. [6]. Mohan B., Rajadurai A., Satyanarayana KG., 2002. Effect of SiC and rotation of electrode on electric discharge machining of Al–SiC composite. J Mater Process Technol 124, 297–304. [7]. Mohan B., Rajadurai A., Satyanarayana KG., 2004. Electric discharge machining of Al–SiC metal matrix composites using rotary tube electrode. J Mater Process Technol, 153–154, 978–985. [8]. Lee CS., Heo EY., Kim JM., Choi IH., Kim DW., 2015. Electrode wear estimation model for EDM drilling. Robot Comput-Integr Manuf, 36, 70–75. [9]. Pradhan BB, Masanta M, Sarkar BR, Bhattacharyya B, 2009. Investigation of electro-discharge micro-machining of titanium super alloy. Int J Adv Manuf Technol, 41/11, 41/12, 1094–1106. [10]. Wang D., Zhao WS., Gu L., Kang XM., 2011. A study on microhole machining of polycrystalline diamond by micro-electrical discharge machining. J Mater Process Technol, 211/1, 3–11. [11]. Pandya S., Menghani J., 2018. Developments of mathematical models for prediction of tensile properties of dissimilar AA6061-T6 to Cu welds prepared by friction stir welding process using Zn interlayer. Sadhana, 43/10, 1-18. [12]. Mohanty CP., Satpathy MP., Mahapatra SS., Singh MR., 2018. Optimization of cryo-treated EDM variables using TOPSIS-based TLBO algorithm. Sadhana, 43/4, 1-18. [13]. Liu GP., Han X., Jiang C., 2012. An efficient multi-objective optimization approach based on the micro genetic algorithm and its application. Int, J, Mech, Mater, Des, 8/1, 37-49. [14]. Santosh T., Patrick K., Georges F., Kalyanmoy D., 2008. An archive based micro genetic algorithm for multi-objective optimization. Proceedings of Genetic and Evolutionary Computation conference, Georgia, USA. [15]. Mogilicharla A., Mittal P., Majumdar S., Mitra K., 2015. Kriging Surrogate Based Multiobjective Optimization of Bulk Vinyl Acetate Polymerization with Branching. Mater. Manuf. Process, 30/4, 394-402. THÔNG TIN TÁC GIẢ Trần Văn Tuấn1, Nguyễn Long Hải2, Nguyễn Trung Thành2 1Khoa Cơ khí, Trường Cao đẳng Công nghiệp Quốc phòng 2Khoa Cơ khí, Học viện Kỹ thuật Quân sự
File đính kèm:
toi_uu_hoa_da_muc_tieu_qua_trinh_khoan_xung_cho_vat_lieu_lam.pdf